The Biofoundry
The Biofoundry is an integral part of the operations at DTU Biosustain. While the Biofoundry supports all the research groups of the Center, its overall aim is to help bridge the technological 'valley of death' (Figure 2) and advance selected research projects in developing cell factories for chemical or protein production. Subsequently, these projects can be handed over to the Pre-Pilot Plant for process optimization, scale-up, and the final spin-out or licensing package. To ensure that projects are economically feasible and can have a significant impact on biosustainability, the Biofoundry maintains strong interactions with the Informatics Platform, Sustainable Innovation Office, and the Commercialization/IP Support team.
The Biofoundry is a data-driven technology platform that enables rapid cell factory design for chemical and protein production. It has been established based on a Design-Build-Test-Learn cycle and comprises ten technology teams (Figure 1). Data analysis is a unique element of the Biofoundry. The Design and Learn teams of the Biofoundry develop and use multiple tools and interoperable databases to transform data into biological meaning, while the Build and Test teams deliver the next efficient cell factory using automation, synthetic biology, adaptive laboratory evolution, and physiological characterization. For more information on the capabilities of each team, please refer to the dedicated links at the top of this page.
Leaders of Biofoundry
-
Jochen Förster (Head of Biofoundry, Build & Test)
-
Bernhard Ø. Palsson (Design & Learn)
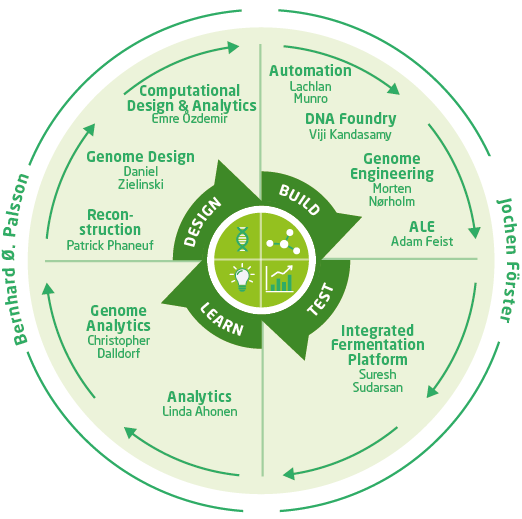
Figure 1. Technology teams in the CFB Biofoundry’s operations
The Biofoundry supports three types of projects:
1. Large Biofoundry Projects. The overall aim is to help bridge the technological "valley of death" (Figure 2) and to advance selected research projects in developing cell factories for chemical or protein production. The intention is to enhance the value of selected research projects and increase their chances for commercial success, e.g., through a spin-off company or attractive licensing agreements with larger enterprises. The research groups at the Center typically work at Technology Readiness Levels (TRLs) 0–2/3 and bring their ideas to a Proof of Concept stage. The Biofoundry provides additional resources to promising projects and supports their continued, rapid development to a first prototype (TRL 4), i.e., a first-generation microbial cell factory. The project can then be transferred to our colleagues at the Pre-Pilot Plant for process optimization and scale-up to TRL 6, culminating in a final spin-out or licensing package (Figure 3).
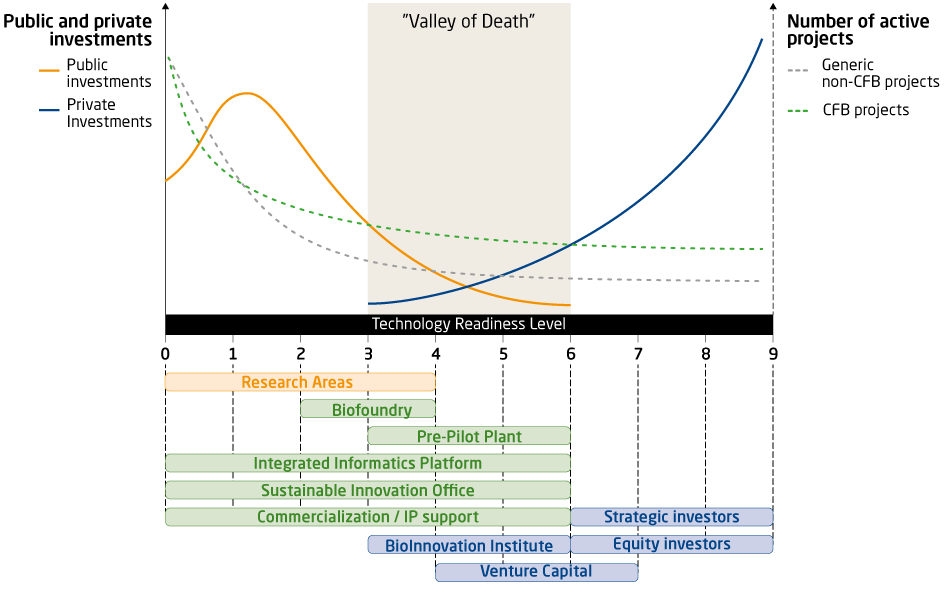
Figure 2. Illustration of CFB’s operations bridging the ‘Valley of Death’.
2. Biofoundry Support Projects. The primary aim is to conduct tasks requested by the Research Groups of our Center, as well as by our sister departments at DTU. We also collaborate with external partners, including universities and industry. Tasks may include omics projects, automation support, and adaptive laboratory evolution projects, etc.
3. Biofoundry Technology Development Projects. Such projects focus on upgrading our technology platform or adding novel technologies that will enable optimized cell factory development.

Figure 3. Research Group + Large Biofoundry Project + Pre-Pilot Plant work together and bring idea to spin-off company.
Contact:
Jochen Förster – jfor@biosustain.dtu.dk