Genome Design Team
The Genome Design team is tasked with deploying well-developed computational tools toward the prospective design and analysis of platform strains. We have previously developed three computational and data analytics tools that are of primary importance for this effort: 1) Metabolic Reconstructions, 2) Genome Sequence Analysis, and 3) Transcriptional Regulatory Network Analysis. We will now assemble a suite of computational tools in the form of the StrainCAD software package, as well as deploying these tools for priority platform strain design projects at the Center to accelerate experimental workflows with computational predictions.
We have two aims:
- Development of a predictive software suite, StrainCAD, for the prospective development of custom-designed genomes.
- Development of genome designs for scale-insensitive strains, C1-utilizing strains, metabolically engineered strains, microbial food strains, and biosynthetic gene cluster expression.
Current capabilities are split into computational and experimental Genome Design (GD) tools. Computational GD capabilities include both modelling and machine learning. Modelling capabilities include constraint-based metabolic modelling, kinetic modelling, thermodynamic modelling. Machine learning capabilities include prediction of thermodynamic and kinetic parameters of reactions, and DNA sequence-based prediction of promoter properties such as expression and regulation. Experimental GD capabilities include iModulon-based strain design, such as iModulon engraftment into new organisms and iModulon engineering via genetic circuits.
Technologies
-
Computational Genome Design
-
gcOpt growth coupling suite
-
Thermodynamic modelling
-
Kinetic Modelling
-
M modelling
-
ME modelling
-
DNA Sequence-based machine learning
-
Experimental Genome Design
-
iModulon engraftment
-
iModulon engineering
Example Work
-
iML1515
-
Amir’s ABC paper
-
Vibrio ICA engineering
Team Member
-
Bernhard Ø. Palsson (Design & Learn)
-
Dan Zielinski (Head of Genome Design)
-
Edward Catoiu, Scientist
-
Amir Akbari, Postdoc
-
Yuan (Annie) Yuan , PhD Student
-
Donghui Choe, Postdoc
-
Jongoh Shin, Postdoc
Selected Publications
1. Sastry AV, Gao Y, Szubin R, Hefner Y, Xu S, Kim D, Choudhary KS, Yang L, King ZA, Palsson BO. The Escherichia coli transcriptome mostly consists of independently regulated modules. Nat Commun. 2019 Dec 4;10(1):5536. doi: 10.1038/s41467-019-13483-w. PMID: 31797920; PMCID: PMC6892915.
2. Akbari A, Yurkovich JT, Zielinski DC, Palsson BO. The quantitative metabolome is shaped by abiotic constraints. Nat Commun. 2021 May 26;12(1):3178. doi: 10.1038/s41467-021-23214-9. PMID: 34039963; PMCID: PMC8155068.
3. Catoiu EA, Phaneuf P, Monk J, Palsson BO. Whole-genome sequences from wild-type and laboratory-evolved strains define the alleleome and establish its hallmarks. Proc Natl Acad Sci U S A. 2023 Apr 11;120(15):e2218835120. doi: 10.1073/pnas.2218835120. Epub 2023 Apr 3. PMID: 37011218; PMCID: PMC10104531.
4. Shin J, Rychel K, Palsson BO. Systems biology of competency in Vibrio natriegens is revealed by applying novel data analytics to the transcriptome. Cell Rep. 2023 Jun 27;42(6):112619. doi: 10.1016/j.celrep.2023.112619. Epub 2023 Jun 6. PMID: 37285268.
5. Anand A, Patel A, Chen K, Olson CA, Phaneuf PV, Lamoureux C, Hefner Y, Szubin R, Feist AM, Palsson BO. Laboratory evolution of synthetic electron transport system variants reveals a larger metabolic respiratory system and its plasticity. Nat Commun. 2022 Jun 27;13(1):3682. doi: 10.1038/s41467-022-30877-5. PMID: 35760776; PMCID: PMC9237125.
Software / Databases:
- Kinetic model construction: MASSef
- Kinetic modelling simulation workflows: MASSpy:
- Online short course on GD and GA tools: Link
- Independent Component Analysis of Prokaryotic Gene Expression: iModulonDB
- Metabolic Model Database: BiGG
Figure 1. Overview of MASSpy features. (A) MASSpy expands COBRApy to provide constraint-based methods for obtaining flux states. (B) Thermodynamic principles are utilized by MASSpy to sample concentration solution spaces and to evaluate how thermodynamic driving forces shift under different metabolic conditions. (C) MASSpy enables dynamic simulation of models to characterize transient dynamic behaviour and contains ensemble modelling methods to represent biological uncertainty. (D) Network properties such as relevant timescales and system stability are characterized by MASSpy using various linear algebra and analytical methods. (E) MASSpy contains built-in functions that enable the visualization of dynamic simulation results. (F) Mechanisms of enzymatic regulation are explicitly modelled in MASSpy through enzyme modules, enabling computation of catalytic activities and functional states of enzymes.

Figure 2. Experimental strain design via iModulon engineering. (A)The process starts with Independent Component Analysis (ICA) to find and classify independently modulated sets of genes (iModulons) using a transcriptome database. (B) Subsequently, a series of synthetic genetic circuits are applied to manipulate iModulon activity in the cells in four different ways. This reprogramming aims to augment specific phenotypes or to tailor cell functions according to desired objectives.
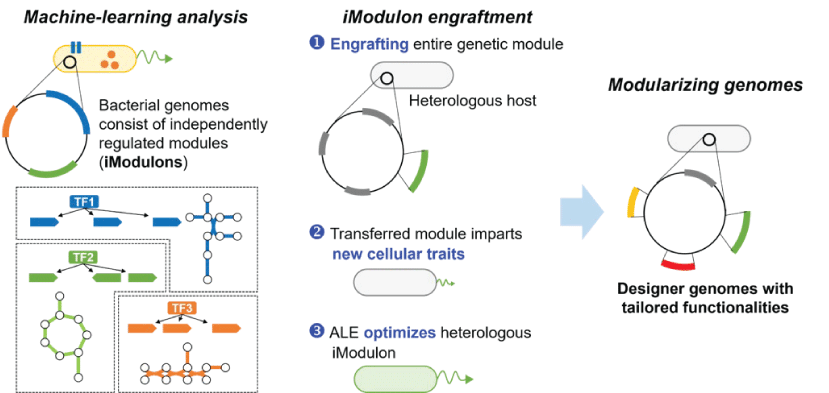
Figure 3. iModulon engraftment provide a rapid way to reconstruct cellular traits. iModulon can be transferred to a heterologous host with single transformation, providing new cellular trait to the recipient. Adaptive laboratory evolution (ALE) induces changes required to accommodate heterologous function. A synthetic genome can be designed and build based on the genomic modules.
Contact:
Dan Zielinski – dczielin@ucsd.edu